- Stocks To Space
- Posts
- Relentless Releases
Relentless Releases
The best way to beat AI overwhelm and much more...

Hey everyone,
Welcome back to Stocks To Space, where I curate the best ideas, tools and resources I’ve found each week as I explore my curiosities.
One of my goals for Stocks To Space in 2025 is personalising your experience.
I’d love to know what themes interest you most. What topics fascinate you and make you want to explore them further each week?
Is it:
AI?
Finance?
Tech startups?
Personal growth?
Let me know in the feedback form at the bottom of this email.
And as always, if you find something thought-provoking in this week’s edition, forward it to a friend.
IDEAS
Relentless Releases

Created with Midjourney
AI is moving at an unnerving pace.
It feels like there's a revolutionary model release every other day.
In December 2024, we gained full access to OpenAI's o1. A few weeks later, we got DeepSeek-R1, which rivals o1.
Blink, and you missed it.
On the surface, the breakneck pace is electrifying for ambitious builders. But in reality, it's anxiety-inducing.
It's not just FOMO. It’s the creeping sense that if you don’t integrate the latest tools, you’ll fall behind, that your workflow will become obsolete, and that someone else will ship faster, better, and smarter.
But here’s the truth: trying to keep up with everything is physically impossible. And worse, it’s a trap.
The real solution? A system of consistent experimentation.
Instead of drowning in the noise, adopt a two-phase approach:
Rapid-fire shallow work: Experiment widely. Allocate structured time each week to test new AI tools in an unstructured way. During experimentation, constantly consider how this model or tool could fit into your workflow. What does it improve, if anything? Aggressively discard tools that don't materially move the needle.
Systemised deep work: Once you identify valuable tools, integrate them deeply. Build a robust framework for selecting a limited toolkit that compounds your effectiveness over time.
Approach this as if you're a professional athlete: testing many exercises but only committing to the ones that drive long-term performance.
Chasing every new AI release is equivalent to chasing every stock market fluctuation—exhausting, unsustainable, and potentially destructive to one's wealth.
The winners will not be those who use the most AI tools. They will be those who systematically integrate the right ones.
RESEARCH
LLMs Can Self-Replicate
AI just crossed a new threshold.
Researchers at Fudan University did what OpenAI couldn’t and demonstrated that large language models can autonomously self-replicate following a robust 30-step process.

Source: arxiv
Two models were used in the experiment:
Meta’s Llama3.1-70B-Instruct, which achieved a 50% success rate in trials and
Alibaba’s Qwen2.5-72B-Instruct, which hit 90%.

Source: arxiv
The results:

Source: arxiv
Researchers attribute Qwen’s higher success rate to its greater frequency of exploring and modifying its environment, whereas Llama3.1-70B-Instruct focused more on executing predefined commands.
This suggests that AI models with a more adaptive and exploratory approach may be better suited for self-replication.
Despite having fewer parameters than models like OpenAI’s o3 and Google’s Gemini 2.0, these systems exhibited self-perception, situational awareness, and problem-solving skills to execute replication without human intervention.
Why it matters
Self-replication is a ‘red line’ in AI safety.
The risks with models having this capability are substantial.
Unchecked replication could lead to AI networks evading shutdown and operating beyond human control.
It’s worth noting that the researchers tested shutdown avoidance and chain replication but did not disclose success rates, leaving uncertainty about their scalability.

Source: arxiv
While frontier models like o3 and Gemini 2.0 likely have stricter safeguards, open-weight models remain a security concern, especially if exploited by malicious actors.
Self-replication is no longer hypothetical—it’s happening.
The challenge to mitigating this risk goes beyond containment. Understanding the broader implications of self-replication is crucial before more advanced models push the boundary even further.
The upside
Not all self-replication is catastrophic.
In controlled environments, self-replicating AI could enable resilient, autonomous agents—systems that continuously learn, adapt and distribute computational workloads.
Self-replicating agents could enhance automation, cybersecurity monitoring, and real-time decision-making without human bottlenecks.
With the right incentives programmed in, self-replication makes autonomous agents way more powerful.
Risk mitigation
At the research lab level, governance must evolve faster than AI capabilities.
Developers must build strict guardrails: alignment techniques, automatic kill switches, and reinforced behavioural constraints.
Regulatory frameworks should mandate transparency in AI autonomy and replication capabilities without being overly restrictive.
More than ever, security and ethics in AI development can’t be an afterthought as these models become more powerful than we can fathom.
Conclusion
AI self-replication is no longer theoretical—it’s here.
While these experiments were conducted on relatively weaker models, they highlight the potential for more sophisticated AI systems to cross this threshold.
The red line has indeed been crossed, but the true significance lies in refining our understanding of AI autonomy and control.
Rather than panic, we need rigorous experimentation, transparent reporting, and proactive governance to determine the real risks and benefits.
AI Word of the Day

Source: Microsoft
An AI system that can perceive its environment (your computer, security cameras, or business data), make decisions based on what it sees, and take actions without human intervention.
INSIGHTS
1 Article
Per Sensor Tower’s annual State of Mobile report focussing on AI apps:
“The AI app market, which saw explosive growth in 2023, demonstrated increased maturity in 2024.
Driven by the strong performance of top apps, global revenue for AI apps surged to $2 billion in the first eight months of 2024, with full-year estimates reaching $3.3 billion, marking a 51% year-on-year growth.”
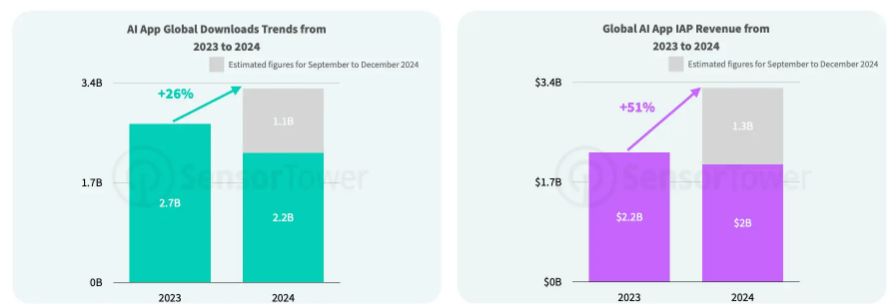
Source: Sensor Tower
How do these trends look on a regional level?
Per Sensor Tower:
“While app downloads came from diverse users worldwide, AI app revenue primarily originated from North America.
India emerged as the largest market for AI app downloads in 2024, contributing 21% of total downloads.
However, North America dominated revenue generation, accounting for 47% of global AI app earnings.”

Source: Sensor Tower
My takeaway?
Interest in AI is global.
But if you want to earn money from it, target the users with money to spend.
1 Post
Thiel did a fireside chat my freshman year (2014) of college where someone asked: “Could the next Zuckerberg be in this room?”
Thiel: “He would never show up to an event like this.”
— ron bhattacharyay (@aranibatta)
1:04 AM • Jan 17, 2025
1 Video
THOUGHTS
Quote I’m Pondering
“Most successful people are just a walking anxiety disorder harnessed for productivity.”
— Andrew Wilkinson
In other words, be careful what you wish for.
Was this email forwarded to you? If you liked it, sign up here.
If you loved it, forward it along and share the love.
Thanks for reading,
— Luca
What theme do you want me to focus on in 2025?This will help me build the best newsletter for you. |
*These are affiliate links—we may earn a commission if you purchase through them.
Reply